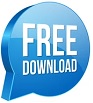
The first is Gauss noise, which belongs to the category of electronic noise that is produced by a sensitive element caused by the random thermal motion of the electronic components. The image noise is divided into three main categories. In the early stage, the traditional de-noising method has a low pass filter method, which mainly includes median filtering, linear filtering and adaptive filtering.ĭuring image collection, coding and transmission, all images are visible or invisible to varying degrees of noise. In recent years, with the deepening of the intersection and research, along with the application of mathematics and other disciplines, the application of fuzzy mathematics, mathematical morphology, intelligent optimization, neural network, and wavelet theory and technology in image processing, as well as some new methods of noise resistance have emerged. Thus, its application is also very extensive. Wavelet transform has a time-frequency local analysis function, and its de-noising results are relatively good.
Wavelet denoise how to#
How to filter out the noise in the real signal to obtain effective information, is a current research hotspot.
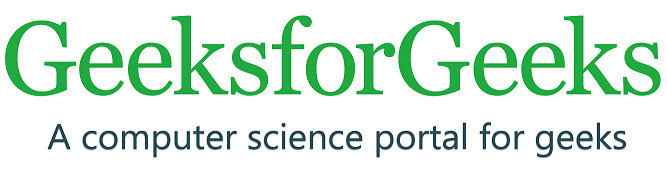
Thus, the implementation of signal de-noising is necessary. The transmission, detection and collection of signals are subject to pollution of varying degrees of random noise, influenced by the environment and due to the nature of the work. Experiment results show that this method, discussed in this paper, is better than traditional hard threshold de-noising and soft threshold de-noising methods, in terms of objective effects and subjective visual effects. Finally, the de-noised images are obtained to rebuild the images in accordance with the estimation in the wavelet-based conditions. Second, the wavelet coefficient is applied on the high-frequency part of the threshold processing by using the improved threshold function. First, the method decomposes the noise image to determine the wavelet coefficients. To address these shortcomings, a method for removing image noise is proposed in this paper. A hard threshold function is discontinuous, whereas a soft threshold function causes constant deviation. Traditional threshold functions have some deficiencies in image de-noising. De-noising methods based on wavelet transform is proposed and achieved with good results, but shortcomings still remain. The wavelet transform in application mathematics has a rapid development. For the characteristics of its multi-analysis, relativity removal, low entropy, and flexible bases, the wavelet transform has become a powerful tool in the field of image de-noising. Therefore, image noise reduction processing is necessary to obtain higher-quality images. However, images are vulnerable to noise interference during collection, transmission and storage, thereby decreasing image quality. With the development of communication technology and network technology, as well as the rising popularity of digital electronic products, an image has become an important carrier of access to outside information. 4School of Nursing, Hong Kong Polytechnic University, Kowloon, Hong Kong.3School of Information and Engineering and Information Technology Centre, WenZhou Medical University, WenZhou, China.2The Chinese People's Liberation Army 118 Hospital, WenZhou, China.

1School of Information and Engineering, The First Affiliated Hospital of WenZhou Medical University, WenZhou Medical University, WenZhou, China.Yang Zhang 1 *, Weifeng Ding 2, Zhifang Pan 3 and Jing Qin 4
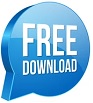