
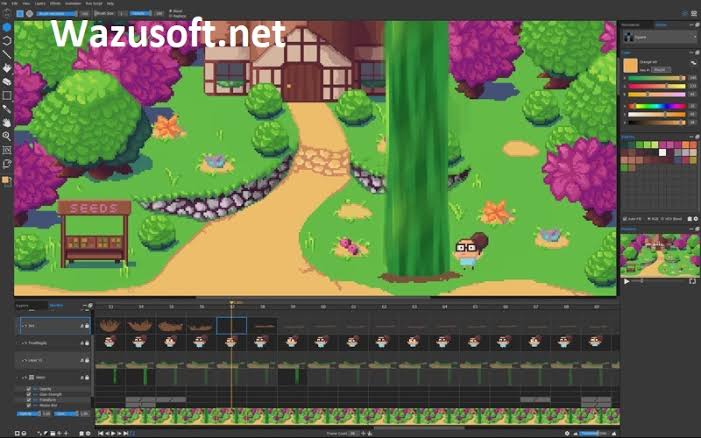
The proposed algorithm uses energy minimization technique to find (near) optimal solution. In this paper, we consider the binary tomography reconstruction problem of images on the hexagonal grid. Therefore, good solutions proposed in continuous space, like total variation (TV) type regularization, are often adapted in discrete space of digital images. The tomography reconstruction problem on continuous domain can be considered as an inverse Radon transform problem, which is, in general case, ill-posed. Numerical experiments on both synthetic and real-world data sets are conducted and demonstrate the validity of our theoretical results and the superiority of our algorithms. Under this quantization scheme, two iterative recovery algorithms are proposed which establish recovery errors decaying at the rate of exponent of the oversampling factor, i.e., exp(−O(λ)). In order to obtain faster decay rate, we introduce a recursive strategy and allow the dithers in quantization to be adaptive to previous measurements for each iterations. As we will see, under the nonadaptive measurement scheme, the recovery errors of two reconstruction procedures decay at the rate of polynomial of the oversampling factor λ := m/(n 1 + n 2)n 3 r, i.e., O(λ −1/6) and O(λ −1/4), respectively. In the case nonadaptive dither exists in the measurements, it is proved that both the direction and the magnitude of X can be simultaneously recovered. In the case no random dither exists in the measurements, our research shows that the direction of tensor X ∈ R n1×n2×n3 with tubal rank r can be well approximated from Ω((n 1 + n 2)n 3 r) random Gaussian measurements.

Two types of recovery models are considered, one is the tensor hard singular tube thresholding and the other one is the tensor nuclear norm minimization. This paper focuses on the recovery of low-tubal-rank tensors from binary measurements based on tensor-tensor product (or t-product) and tensor Singular Value Decomposition (t-SVD). Based on the performance comparison of a number of test images, we can say that the new method outperforms the energy minimization-based denoising methods often used in the literature for method comparison. The experiments provided in this paper confirm the capability of the proposed approach for providing high-quality reconstructions. This operator is the shape elongation measure, one of the shape descriptors intensively used in shape-based image processing and computer vision tasks. it also expresses the intensity variation in a neighborhood of the considered point), but is concurrently applicable in both continuous and discrete space. In this paper we propose a new approach, where the gradient magnitude function is replaced with an operator with similar properties (i.e. when dealing with digital images), the accuracy in the gradient computation is limited by the applied image resolution. The total variation, comprising the gradient magnitude function, originally comes from mathematical analysis and is defined on a continuous domain only. A common approach to denoising images is to minimize an energy function combining a quadratic data fidelity term with a total variation-based regularization.
